Table of Contents
Introduction
Scaling AI has swiftly transitioned from a futuristic vision to a driving force behind technological advancements across various industries. Its potential to revolutionize business operations, streamline processes, and create unparalleled customer experiences is undeniable. Today, AI is not just about innovation but also about reshaping the way industries function. However, for AI to truly scale and realize its full potential, organizations must confront several significant challenges. These include ensuring the quality and quantity of data, addressing ethical dilemmas, navigating the talent shortage, and managing the costs of infrastructure and deployment.
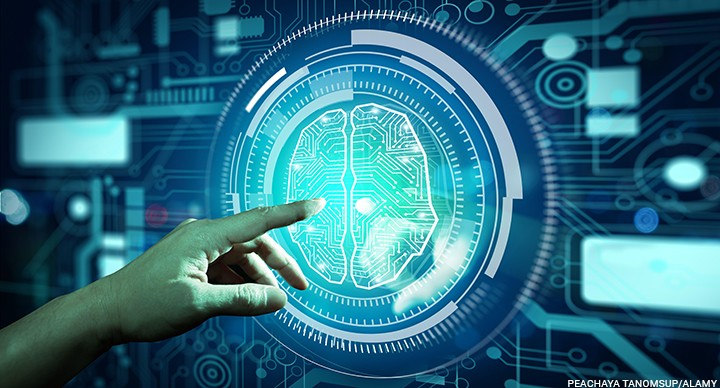
Challenges Hindering Scaling AI
Scaling AI comes with a host of challenges that can hinder its full potential. These include:
- Data Quality and Quantity: AI models thrive on large volumes of high-quality, well-labeled data for effective learning. However, many organizations struggle to collect, curate, and maintain datasets that are both robust and representative. Poor data can result in biased, inaccurate, or unreliable AI predictions, which can adversely affect business decisions. Moreover, acquiring high-quality, domain-specific data is often time-consuming and costly. Without resolving these data challenges, scaling AI initiatives is an uphill battle.
- Ethical Considerations: The rapid deployment of AI technologies raises important ethical issues, particularly in areas such as privacy, bias, and transparency. AI systems can inadvertently amplify biases present in training data, leading to unfair or discriminatory outcomes. Additionally, there are growing concerns about privacy violations and the accountability of AI decision-making processes. For AI to scale successfully, organizations must prioritize creating systems that are not only technically proficient but also fair, transparent, and aligned with ethical standards.
- Talent Shortage: As AI adoption grows, the demand for skilled professionals outpaces the supply. Organizations worldwide face difficulty in finding talent with the necessary expertise in AI development, implementation, and maintenance. Roles such as data scientists, machine learning engineers, and AI ethicists are in high demand, but the talent pool remains limited. This talent gap hinders organizations from fully embracing and scaling AI solutions.
- Infrastructure and Cost: Implementing AI at scale requires a significant investment in computational resources, infrastructure, and tools. AI models, especially those powered by deep learning, often require powerful GPUs, large-scale cloud computing services, and specialized software frameworks. The costs associated with these resources can be prohibitively high for many organizations, particularly small to mid-sized businesses. Additionally, managing and maintaining AI infrastructure can be complex and costly over time.
How AI Can Help in Industry Innovation
Despite the challenges, AI offers substantial opportunities for industries to innovate, optimize operations, and deliver value:
- Process Optimization: One of AI’s greatest strengths is its ability to automate repetitive tasks and optimize processes. By analyzing patterns and inefficiencies within workflows, AI-driven solutions can enhance productivity and reduce costs. For instance, AI-powered automation can streamline supply chains, improve inventory management, and optimize resource allocation in real-time.
- Personalized Customer Experiences: AI enables businesses to harness the power of data to deliver hyper-personalized customer experiences. From personalized product recommendations to chatbots that provide real-time assistance, AI enhances customer satisfaction and drives sales. In retail and e-commerce, AI can analyze purchasing behaviors to tailor marketing campaigns and product offerings, leading to more engaged customers and higher conversion rates.
- Product Development: AI accelerates product development by simulating testing environments, analyzing user data, and identifying market trends. It can streamline design processes, reduce time-to-market, and highlight emerging opportunities. For example, AI can predict consumer preferences, which allows companies to create products that better meet market demands.
- Decision Making: AI-powered analytics provide organizations with actionable insights that aid decision-making. From predictive models to real-time recommendations, AI helps businesses make informed decisions, minimizing risks and improving outcomes. Whether it’s optimizing business strategies or forecasting demand, AI enables leaders to make smarter, data-driven choices.
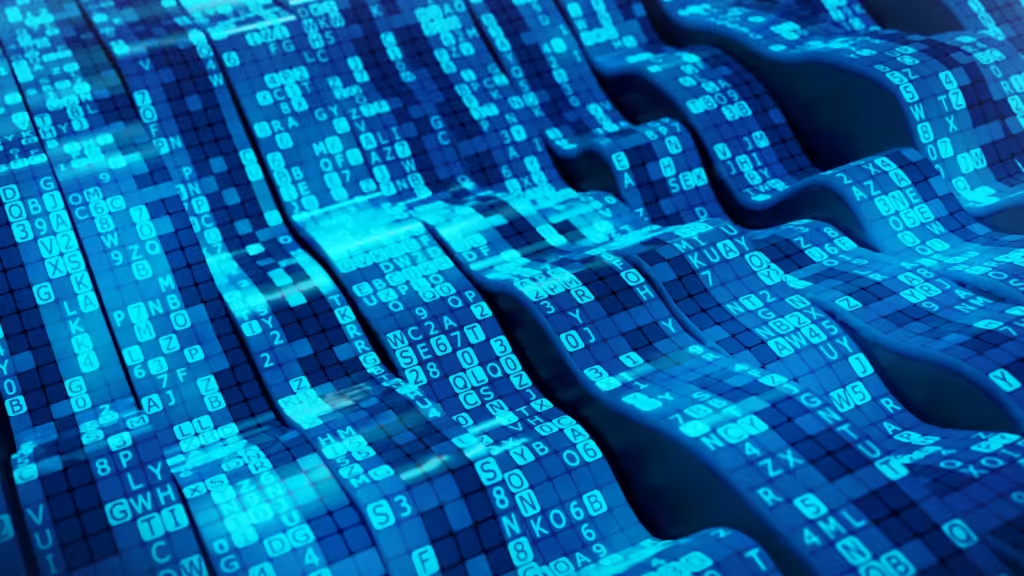
How AI Affects the Growth of Different Industries
AI’s transformative potential is evident across various sectors, each benefiting uniquely from its applications:
- Healthcare: AI is revolutionizing healthcare by making diagnoses more accurate, creating personalized treatment plans, and advancing drug discovery. AI-driven tools can analyze medical data faster and with greater precision than traditional methods, improving patient outcomes. Predictive analytics in healthcare can help doctors anticipate patient needs, and AI algorithms can discover potential new drugs by analyzing vast datasets of molecular compounds.
- Manufacturing: In manufacturing, AI is being used to automate production lines, implement predictive maintenance, and improve quality control. AI-driven automation not only enhances operational efficiency but also reduces human error, leading to fewer defects and lower operational costs. Predictive maintenance helps in preventing machinery breakdowns by forecasting potential failures, which results in reduced downtime and maintenance costs.
- Finance: The finance industry has embraced AI for fraud detection, risk management, and algorithmic trading. AI-powered algorithms analyze vast amounts of transactional data to detect suspicious patterns, preventing fraud before it escalates. Additionally, AI tools assist in making investment decisions by analyzing market trends and assessing risk with unparalleled accuracy, helping financial institutions improve services while mitigating risks.
- Retail: AI enhances the retail experience through personalized marketing, efficient inventory management, and superior customer service. Retailers use AI-driven tools to analyze customer preferences and purchase history, allowing them to tailor marketing efforts to individual consumers. AI also optimizes stock levels by forecasting demand, preventing overstocking and stockouts.
Conclusion
While the journey to scaling AI comes with significant challenges—ranging from data quality issues and ethical considerations to infrastructure costs and a shortage of skilled talent—overcoming these hurdles can lead to extraordinary benefits. AI has the potential to revolutionize industries by driving innovation, optimizing processes, and transforming customer experiences. As organizations continue to adopt AI, addressing these challenges head-on will be crucial for realizing the full potential of this transformative technology and achieving long-term, sustainable growth.
What are the key challenges in scaling AI?
The main challenges include data quality and availability, ethical concerns such as privacy and bias, the shortage of skilled AI professionals, and the high costs of infrastructure required to support AI solutions. These factors can limit the widespread adoption and effective scaling of AI technologies.
Why is scaling AI expensive?
Scaling AI requires significant investments in computational resources, including powerful hardware like GPUs, specialized software, and cloud infrastructure. In addition, maintaining these resources and managing large datasets can be costly, especially for smaller organizations. However, cloud-based AI platforms and more affordable AI tools are helping to reduce these costs.
Why is data quality so important for AI scaling?
High-quality, accurate, and well-labeled data is crucial for training AI models. Poor data can lead to biased or incorrect outputs, reducing the effectiveness of AI systems. Reliable data ensures that AI models can learn effectively and produce useful insights.