Introduction
As artificial intelligence (AI) continues to advance, its growing computational demands have raised concerns about energy consumption. AI models, particularly deep learning architectures, require substantial processing power and energy, leading to an energy crisis that many experts predict will hinder the future of AI development. However, emerging technologies like quantum computing may hold the key to alleviating this energy crisis. This blog delves into the interplay between quantum computing and AI, exploring how quantum innovations could transform AI’s energy landscape, alongside insights from notable figures like Elon Musk.
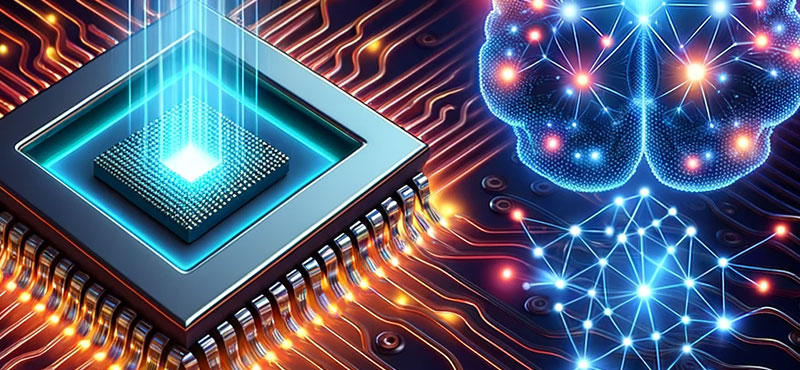
Can Quantum Computing Help AI?
Quantum computing leverages the principles of quantum mechanics to perform calculations at unprecedented speeds and efficiencies. Unlike classical computers, which process information in binary (0s and 1s), quantum computers use qubits, which can exist in multiple states simultaneously. This capability allows quantum computers to solve complex problems that would take classical computers an impractical amount of time and energy.
1. Optimizing AI Algorithms
Quantum computing can potentially revolutionize AI by optimizing algorithms that are currently energy-intensive. Many machine learning algorithms, particularly those used in training deep neural networks, require massive amounts of data and computational resources. Quantum algorithms, such as the Quantum Approximate Optimization Algorithm (QAOA) and Quantum Support Vector Machines (QSVM), can significantly reduce the computational complexity of these processes.
For instance, a study published in Nature highlights how quantum algorithms can optimize the training of neural networks, reducing the time and energy needed for large-scale model training. By enabling faster convergence to optimal solutions, quantum computing could dramatically lower the energy required for AI model training and inference.
2. Data Handling and Processing
AI relies on vast amounts of data, and the ability to process this data efficiently is crucial. Quantum computers excel in handling large datasets due to their unique ability to represent and manipulate complex probability distributions. Quantum-enhanced data processing can lead to more efficient data analysis, enabling AI systems to learn from data more effectively while consuming less energy.
For example, researchers are exploring quantum machine learning algorithms that utilize quantum states to encode data, allowing for faster processing and improved accuracy. This shift could mean that AI systems become capable of training on larger datasets in significantly less time, ultimately reducing their overall energy consumption.
3. Solving Complex Problems
AI is often employed to solve complex problems in various fields, such as healthcare, finance, and logistics. Quantum computing’s ability to tackle NP-hard problems efficiently can enhance AI’s problem-solving capabilities. By using quantum algorithms, AI systems can explore a more extensive solution space quickly, leading to more efficient outcomes.
A notable case study involves quantum computing’s application in optimizing supply chain logistics. Quantum computers can analyze various logistical parameters simultaneously, leading to more efficient routing and inventory management solutions. This application not only improves operational efficiency but also reduces the energy consumed in transportation and storage.
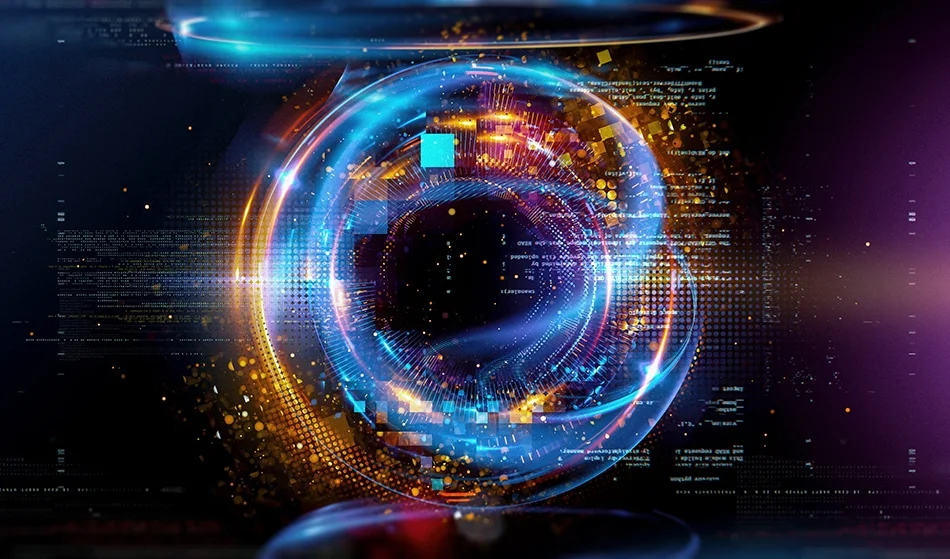
How Can AI Solve the Energy Crisis?
While quantum computing presents a promising avenue for reducing AI’s energy demands, AI itself can also play a role in addressing the energy crisis.
1. Energy Management Systems
AI technologies are increasingly being integrated into energy management systems, helping to optimize energy consumption in real time. These systems leverage machine learning algorithms to predict energy demand patterns, allowing for more efficient energy distribution and reduced waste.
For example, Google has implemented AI in its data centers to optimize cooling systems. By analyzing temperature and usage data, the AI system can dynamically adjust cooling settings, leading to a 40% reduction in energy usage for cooling, which represents a significant portion of a data center’s energy consumption.
2. Smart Grids
AI is a key component of smart grid technology, which aims to create a more resilient and efficient energy infrastructure. By analyzing data from various sources, AI can predict energy demand, identify faults, and optimize energy distribution. This capability can lead to more effective integration of renewable energy sources, further reducing reliance on fossil fuels.
Case studies from cities that have adopted smart grid technology, such as San Diego, demonstrate substantial energy savings and improved grid stability. By utilizing AI to manage energy flow, these cities have reported significant reductions in energy waste and improved utilization of renewable energy sources.
3. Predictive Maintenance
AI-driven predictive maintenance can also contribute to energy savings. By analyzing sensor data from machinery and infrastructure, AI can predict when equipment is likely to fail or become less efficient. This foresight allows for timely repairs and adjustments, preventing energy waste caused by malfunctioning equipment.
Industries such as manufacturing and transportation have reported notable energy savings by adopting predictive maintenance strategies. For example, GE Aviation uses AI to predict engine maintenance needs, leading to reduced downtime and improved fuel efficiency in aircraft operations.
What Does Elon Musk Think About Quantum Computing?
Elon Musk, a prominent entrepreneur and tech visionary, has expressed both interest and skepticism regarding quantum computing. Musk recognizes the potential of quantum computing to revolutionize various sectors, including AI, but also emphasizes the challenges and limitations currently facing the technology.
1. Potential Applications
In interviews, Musk mentioned that quantum computing could accelerate advancements in AI, particularly in enhancing AI’s ability to process information and solve complex problems. He acknowledges that quantum computers could outperform classical computers in specific tasks, making them valuable for AI research and development.
2. Skepticism About Practicality
Despite his recognition of quantum computing’s potential, Musk has also voiced concerns about its practical implementation. He points out that quantum computers are still in their infancy, and significant advancements are needed before they can be widely adopted. Musk’s caution is rooted in the belief that many quantum systems are still plagued by issues such as qubit coherence and error rates, which hinder their practical use.
3. Focus on AI Safety
Musk is known for his emphasis on AI safety and ethical considerations surrounding AI development. He believes that the rapid advancements in AI, including those potentially fueled by quantum computing, require careful oversight and regulation to prevent unintended consequences. Musk’s views suggest that while quantum computing could offer solutions to AI’s energy challenges, the ethical implications of these technologies must also be considered.
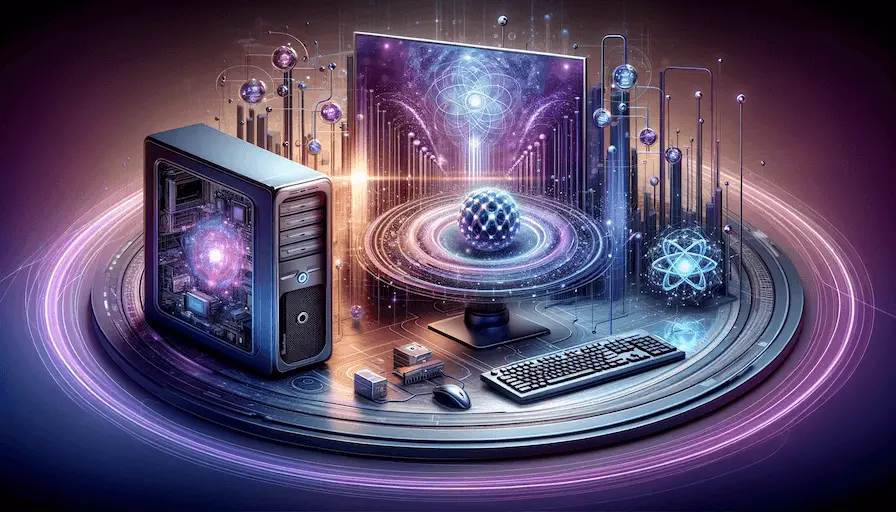
Case Studies Illustrating the Interplay Between Quantum Computing and AI
1. IBM Quantum and AI
IBM has been at the forefront of integrating quantum computing with AI. Their research explores how quantum algorithms can enhance machine-learning tasks. A notable case study involved using IBM’s quantum computer to optimize a machine learning model for drug discovery, demonstrating how quantum computing can expedite complex simulations and reduce the energy footprint associated with traditional methods.
2. D-Wave Systems
D-Wave Systems, a leader in quantum computing, has collaborated with various organizations to apply quantum technology to AI challenges. One example is their partnership with Volkswagen to optimize traffic flow in urban areas using quantum computing. The project aimed to reduce congestion and energy consumption in cities, illustrating how quantum computing can address real-world energy challenges through AI applications.
3. Google’s Quantum AI Lab
Google’s Quantum AI Lab is actively researching the intersection of quantum computing and AI. Their work includes developing quantum algorithms that can accelerate machine learning tasks, such as pattern recognition and natural language processing. A significant outcome of their research is the demonstration of a quantum algorithm that can outperform classical counterparts in specific machine learning tasks, highlighting the potential for reduced energy consumption in AI applications.
Conclusion
As AI continues to evolve and face mounting energy challenges, quantum computing emerges as a potential solution that could revolutionize the field. By optimizing algorithms, enhancing data processing capabilities, and solving complex problems, quantum computing can significantly reduce the energy demands of AI systems. Simultaneously, AI technologies can contribute to addressing the broader energy crisis through efficient energy management and predictive maintenance strategies.
While insights from tech leaders like Elon Musk emphasize both the promise and challenges of quantum computing, ongoing research, and real-world applications illustrate the potential synergy between these two transformative technologies. As we explore this intersection, it becomes clear that the future of AI and quantum computing could pave the way for a more sustainable and energy-efficient world.
By harnessing the power of quantum computing, we may not only solve AI’s energy crisis but also unlock new possibilities for innovation across various sectors.
What is the future of quantum computing and AI?
The future of quantum computing and AI looks promising, with ongoing research and development aiming to enhance their synergy. As quantum technology matures, it may significantly impact AI applications, leading to more efficient algorithms and reduced energy consumption in various sectors.
What role do smart grids play in the intersection of AI and energy management?
Smart grids use AI to analyze data and optimize energy distribution in real time. They enable better integration of renewable energy sources, predictive maintenance of infrastructure, and overall improved energy efficiency, helping to mitigate the energy crisis.
How can quantum computing reduce the energy consumption of AI models?
Quantum computing can reduce energy consumption by enabling faster convergence of machine learning models, optimizing algorithmic complexity, and improving data handling efficiency. This leads to shorter training times and less energy expenditure.
What are some quantum algorithms relevant to AI?
Some notable quantum algorithms relevant to AI include the Quantum Approximate Optimization Algorithm (QAOA) and Quantum Support Vector Machines (QSVM). These algorithms can optimize machine learning processes and enhance AI’s efficiency.
Are there any real-world applications of quantum computing in AI?
Yes, several organizations are exploring the application of quantum computing in AI. For example, IBM has used quantum algorithms to optimize drug discovery processes, and D-Wave Systems collaborated with Volkswagen to improve traffic flow and reduce energy consumption in urban settings.