Table of Contents
AI in healthcare transforming the practice of medicine. In today’s rapidly evolving healthcare landscape, artificial intelligence (AI) is emerging as a powerful catalyst for transformation. With its ability to analyze vast amounts of data, identify patterns, and make intelligent decisions, AI is poised to revolutionize the way we deliver healthcare. This blog post will explore how AI can enhance efficiency and accuracy across various aspects of the healthcare industry, from diagnosis and treatment to administrative tasks.
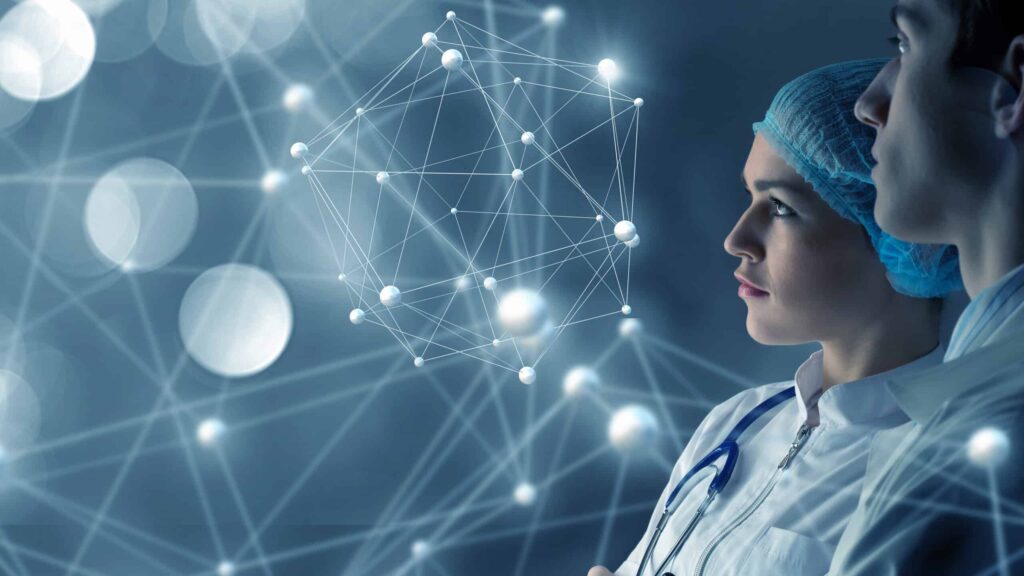
How accurate is AI in healthcare?
AI’s accuracy in healthcare is impressive but not perfect. While it can outperform humans in certain tasks, such as analyzing medical images or predicting patient outcomes, its accuracy depends on the quality and quantity of data it’s trained on. Additionally, AI algorithms can be biased, leading to inaccurate or discriminatory results.
Precision diagnostics AI in healthcare
Diagnostic imaging – AI in Diagnostic Imaging: A Game-Changer. By leveraging advanced algorithms and machine learning techniques, AI can analyze medical images, such as X-rays, CT scans, and MRIs, with unprecedented accuracy and speed. This has significant implications for patient care, diagnosis, and treatment planning.
Key Benefits of AI in Diagnostic Imaging:
- Improved Accuracy: AI can detect subtle abnormalities that may be missed by human radiologists, leading to earlier diagnoses and more effective treatments.
- Increased Efficiency: AI can automate routine tasks, such as image segmentation and classification, freeing up radiologists to focus on complex cases.
- Enhanced Consistency: AI can help to standardize image interpretation, reducing variability between radiologists and improving diagnostic consistency.
- Personalized Medicine: AI can be used to develop personalized treatment plans based on a patient’s specific imaging data and genetic information.
Applications of AI in Diagnostic Imaging:
- Cancer Detection: AI can assist in the early detection of various cancers, including breast, lung, and prostate cancer.
- Cardiovascular Disease: AI can help identify abnormalities in the heart and blood vessels, aiding in the diagnosis and management of cardiovascular diseases.
- Neurological Disorders: AI can be used to detect and monitor neurological conditions such as stroke, Alzheimer’s disease, and multiple sclerosis.
- Orthopedic Conditions: AI can assist in the diagnosis and assessment of orthopedic injuries and diseases.
improving the precision and reducing waiting timings for radiotherapy planning – AI in healthcare is revolutionizing the field of radiotherapy planning, a critical step in cancer treatment. By leveraging advanced algorithms and machine learning techniques, AI can significantly improve the precision and efficiency of this process, ultimately leading to better patient outcomes.
Key Benefits of AI in Radiotherapy Planning:
- Enhanced Precision: AI can analyze patient data, including CT scans and MRI images, with greater accuracy than traditional methods. This allows for more precise targeting of tumors, reducing the risk of damaging healthy tissues.
- Reduced Waiting Times: AI can automate many of the time-consuming tasks involved in radiotherapy planning, such as contouring and dose calculation. This can significantly reduce waiting times for patients, ensuring they receive timely treatment.
- Personalized Treatment Plans: AI can help create highly personalized treatment plans based on a patient’s individual characteristics and tumor biology.
- Improved Treatment Outcomes: By optimizing radiotherapy planning, AI can contribute to improved patient outcomes, including higher survival rates and better quality of life.
Applications of AI in Radiotherapy Planning:
- Adaptive Radiotherapy: AI can be used to adjust treatment plans in real-time based on changes in tumor size or location, ensuring optimal treatment delivery.
- Intensity-Modulated Radiation Therapy (IMRT): AI can help create complex IMRT plans that deliver high doses of radiation to tumors while minimizing damage to surrounding healthy tissues.
- Stereotactic Radiosurgery (SRS): AI can assist in the planning and delivery of SRS treatments for small, localized tumors.
How does AI in Healthcare improve accuracy?
AI improves accuracy in various ways:
- Data Analysis and Pattern Recognition: AI algorithms can analyze vast datasets to identify patterns and trends that humans might miss.
- Automation and Error Reduction: AI can automate tasks that are prone to human error, reducing the likelihood of mistakes and improving accuracy.
- Continuous Learning and Improvement: AI systems can learn from their experiences and adapt over time, improving their accuracy as they encounter new data and situations.
- Objectivity and Bias Reduction: AI can make decisions based on data rather than subjective opinions, reducing the risk of bias and improving accuracy.
- Advanced Algorithms: AI utilizes sophisticated algorithms, such as machine learning and deep learning, that are specifically designed to improve accuracy and performance.
How does AI improve efficiency?
Data Analysis and Pattern Recognition: AI algorithms can analyze vast datasets to identify patterns and trends that humans might miss.
· Automation and Error Reduction: AI can automate tasks that are prone to human error, reducing the likelihood of mistakes and improving accuracy.
· Continuous Learning and Improvement: AI systems can learn from their experiences and adapt over time, improving their accuracy as they encounter new data and situations.
· Objectivity and Bias Reduction: AI can make decisions based on data rather than subjective opinions, reducing the risk of bias and improving accuracy.
Advanced Algorithms: AI utilizes sophisticated algorithms, such as machine learning and deep learning, that are specifically designed to improve accuracy and performance
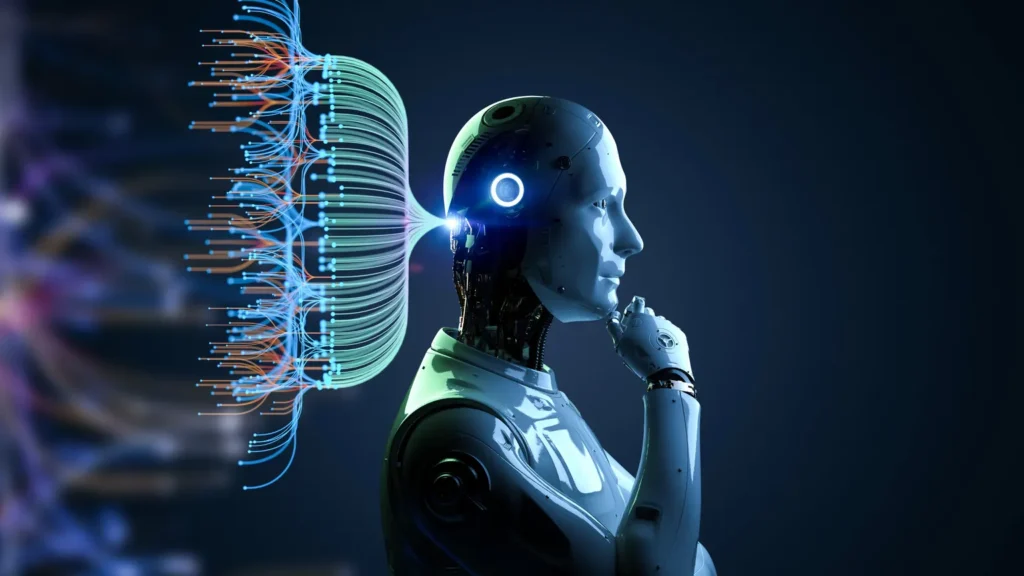
AI-driven drug discovery
Traditional drug discovery methods are time-consuming and expensive, often taking years to bring new drugs to market. AI offers a more efficient and effective approach by leveraging advanced algorithms and machine learning techniques.
Key Benefits of AI-Driven Drug Discovery:
- Faster Development: AI can analyze vast amounts of data, including genetic information, clinical trials, and chemical structures, to identify promising drug candidates more quickly.
- Improved Accuracy: AI can predict the properties of potential drugs, such as efficacy, toxicity, and safety, with greater accuracy than traditional methods. This helps to minimize the risk of failure in clinical trials and reduce development costs.
- Enhanced Target Identification: AI can help researchers identify new drug targets, which are the specific molecules or biological processes involved in diseases.
- Personalized Medicine: AI can be used to develop personalized treatments based on a patient’s individual genetic makeup and disease characteristics. This can solve treatment outcomes and reduce side effects.
Applications of AI in Drug Discovery:
- Target Identification: AI can analyze genetic data to identify new drug targets and predict their potential efficacy.
- Lead Optimization: AI can help optimize the structure of drug molecules to improve their properties, such as potency, selectivity, and bioavailability.
- Virtual Screening: AI can be used to virtually screen millions of compounds to identify potential drug candidates.
- Clinical Trial Design: AI can help design more efficient and effective clinical trials by predicting patient outcomes and optimizing study protocols.
AI empowered healthcare professionals
By providing valuable insights, automating tasks, and enhancing decision-making, AI is empowering healthcare providers to deliver more effective, efficient, and personalized care.
Key Benefits of AI for Healthcare Professionals:
- Enhanced Decision-Making: AI can analyze vast amounts of patient data, including medical records, imaging results, and genetic information, to provide valuable insights that can inform clinical decision-making. This can lead to more accurate diagnoses, more effective treatments, and improved patient outcomes.
- Improved Efficiency: AI can automate many routine tasks, such as data entry, documentation, and scheduling, freeing up healthcare professionals to focus on providing direct patient care.
- Personalized Care: AI can help healthcare professionals develop personalized treatment plans based on a patient’s individual characteristics and medical history. This can help patient outcomes and satisfaction.
- Continuous Learning: AI can facilitate continuous learning by providing healthcare professionals with access to the latest research and best practices. This can help to ensure that they are delivering the highest quality care.
Applications of AI for Healthcare Professionals:
- Diagnostic Support: AI can assist in the diagnosis of diseases by analyzing medical images, interpreting lab results, and identifying patterns in patient data.
- Treatment Planning: AI can help healthcare professionals develop personalized treatment plans based on a patient’s individual needs and preferences.
- Remote Monitoring: AI-powered devices can be used to monitor patients remotely, allowing healthcare professionals to intervene early if necessary.
- Clinical Research: AI can accelerate clinical research by analyzing large datasets and identifying new drug targets.
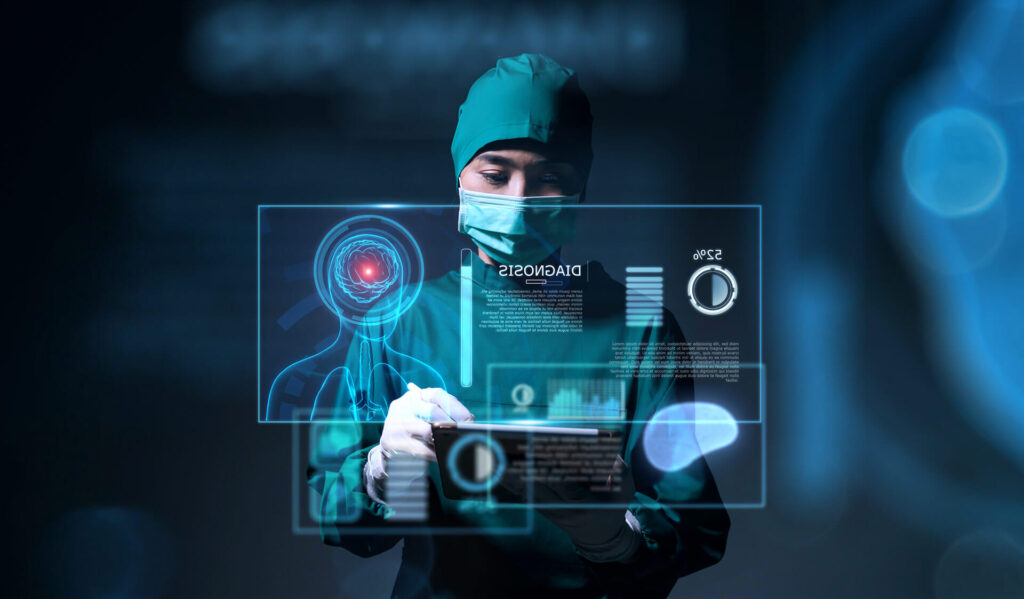
What are the current and future use cases of AI in healthcare?
AI today and in the near future – AI in healthcare is already making significant strides, with applications ranging from drug discovery to patient diagnosis. In the near future, we can expect to see even more widespread adoption of AI-powered tools and technologies. Some key areas of focus include:
- Enhanced diagnostic accuracy: AI in healthcare will continue to improve its ability to analyze medical images, detect abnormalities, and assist in early diagnosis of diseases.
- Personalized treatment plans: AI-driven algorithms will enable the creation of highly tailored treatment plans based on individual patient data, genetics, and disease characteristics.
- Remote patient monitoring: AI-powered devices will enable remote monitoring of patients, allowing for early detection of health issues and more efficient management of chronic conditions.
- Drug discovery and development: AI will accelerate the process of drug discovery and development by analyzing vast amounts of data to identify promising drug candidates and optimize their properties.
AI in healthcare in the next five to ten years, or the medium term-
some of key feathers may include
- Robotic surgery: AI-powered robots will become more sophisticated and capable of performing complex surgical procedures with greater precision and accuracy than human surgeons.
- Wearable health devices: AI-enabled wearable devices will become more advanced, providing real-time monitoring of vital signs, detecting health issues, and offering personalized health recommendations.
- AI-powered medical assistants: AI-driven virtual assistants will be able to provide patients with information, answer questions, and even assist with basic medical tasks.
- Predictive analytics: AI will be used to predict the likelihood of certain health outcomes based on patient data, allowing for early intervention and prevention.
3. AI in healthcare in the Long Term (>10 year)- In the long term
AI has the potential to revolutionize healthcare. Some visionary ideas include:
- AI-driven drug discovery: AI could be used to discover entirely new classes of drugs that are more effective and have fewer side effects.
- Personalized medicine: AI could enable the creation of highly personalized treatments tailored to the specific needs of individual patients, leading to significant improvements in health outcomes.
- Human-AI collaboration: AI could work alongside healthcare professionals to enhance their capabilities and improve patient care.
- Disease prevention: AI could be used to predict and prevent diseases before they occur, leading to a healthier population and reduced healthcare costs.
Challenges for AI in healthcare
1. Data Quality and Quantity
- Data scarcity: Obtaining high-quality, labelled healthcare data can be challenging, especially for rare diseases or specific patient populations.
- Data privacy and security: Ensuring the privacy and security of patient data is crucial, but it can be difficult to balance this with the need for large datasets to train AI models.
2. Algorithmic Bias
- Bias in data: If the data used to train AI models is biased, the models will likely perpetuate that bias, leading to unfair or inaccurate outcomes.
- Algorithmic bias: AI algorithms themselves can be biased, especially if they are not carefully designed and evaluated.
Conclusion
AI in healthcare has the potential to revolutionize healthcare by improving efficiency, accuracy, and patient outcomes. By addressing the challenges and ethical considerations, we can harness the power of AI to create a more equitable, accessible, and effective healthcare system.