Table of Contents
Introduction
The convergence of ML and quantum technology marks a paradigm shift in computational power and intelligence. Quantum computing, a field that harnesses the principles of quantum mechanics to perform calculations far beyond the reach of classical computers, is being supercharged by machine learning algorithms. Together, these technologies open new horizons in everything from optimization problems and drug discovery to secure communications and artificial intelligence.
This blog delves into how machine learning is applied in quantum computing, the quantum theory of machine learning, and the distinct advantages of quantum machine learning (QML). Through use cases and theoretical insights, we will explore how these intertwined domains shape the future of computation.
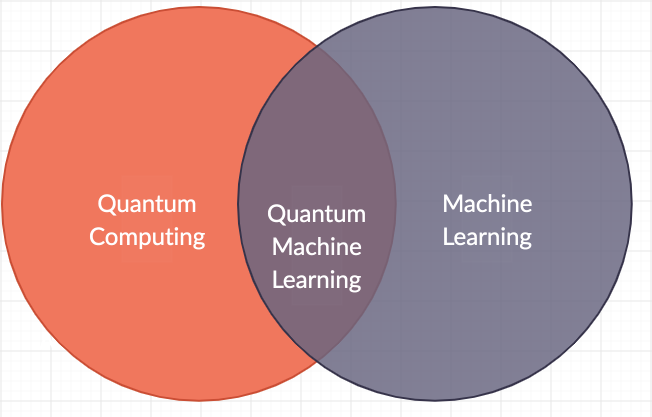
How is Machine Learning Used in Quantum Computing?
1. Enhancing Quantum Algorithms with Machine Learning
Machine learning is integral to the improvement of quantum algorithms, which are the backbone of quantum computing. Unlike classical algorithms that operate sequentially, quantum algorithms like Shor’s algorithm for factoring large integers or Grover’s search algorithm leverage quantum superposition and entanglement. However, optimizing these algorithms for real-world applications is challenging. ML helps by discovering new quantum algorithms, optimizing existing ones, and improving their efficiency, especially in handling noisy quantum states.
2. Noise Reduction and Error Correction
One of the biggest challenges in quantum computing is maintaining qubit coherence (the state in which qubits can perform meaningful calculations) in the face of noise and external interference. ML algorithms have been employed to design effective error correction protocols. By recognizing patterns in quantum data and classifying qubit errors, ML techniques contribute to creating more robust quantum systems.
3. Quantum Data Classification and Optimization
Quantum computing excels at dealing with complex data sets, especially high-dimensional data. ML is used for quantum data classification, allowing quantum systems to recognize patterns that classical systems cannot. This is critical for applications such as cryptography, material science, and climate modeling, where the complexity of the data demands advanced computational power.
4. Quantum Machine Learning for Optimization Problems
Optimization problems are central to many fields, including logistics, finance, and supply chain management. Quantum ML techniques are already being applied to solve large-scale optimization problems more efficiently than classical methods. For example, a quantum support vector machine (SVM) can solve classification tasks much faster, enhancing ML’s impact on various industries.
What is the Quantum Theory of Machine Learning?
1. Introduction to Quantum Machine Learning (QML)
Quantum machine learning blends quantum computing with ML principles to create a new form of computation. The underlying idea is that quantum computers, by leveraging quantum bits or qubits, can process vast amounts of data and perform complex computations more efficiently than classical computers.
Quantum machine learning models, such as quantum neural networks (QNNs), extend the capabilities of classical ML algorithms. In QML, quantum states are manipulated to find solutions that might take classical computers much longer to compute. This is a significant shift because it allows ML algorithms to tackle problems previously considered unsolvable.
2. Hybrid Quantum-Classical Models
One emerging concept in the quantum theory of ML is the hybrid model, where quantum computers handle the data-heavy components of a problem, and classical systems manage the easier, more computationally efficient tasks. These hybrid models take advantage of both quantum speedups and classical simplicity, offering a practical approach for near-term quantum systems that are still prone to errors.
3. Quantum Kernel Methods
A fundamental concept in QML is the quantum kernel method, which uses quantum computers to perform feature space mapping, making it easier to classify complex data patterns. Quantum kernel estimation is believed to outperform classical kernel methods, especially in cases where classical feature spaces are inefficient.
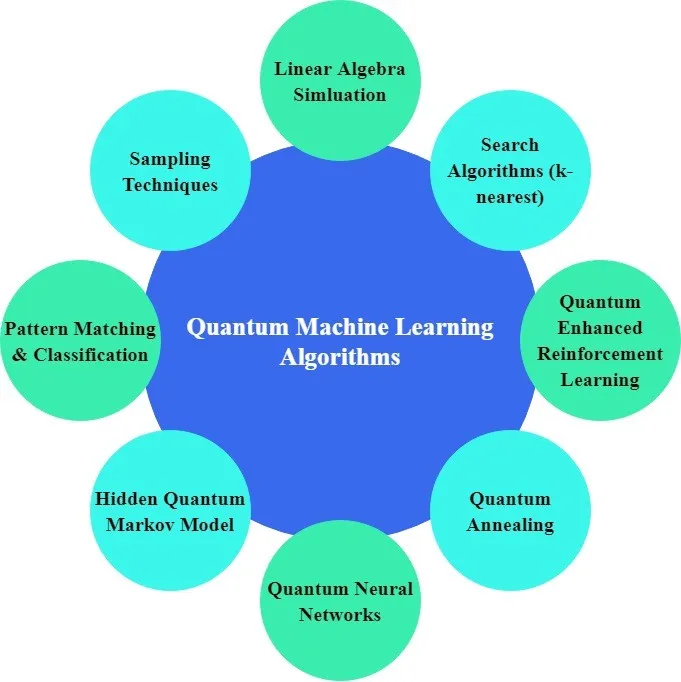
What is the Advantage of Quantum Machine Learning?
1. Exponential Speedup
One of the most discussed advantages of quantum machine learning is its exponential speedup over classical ML algorithms. Quantum computers can evaluate many solutions simultaneously, thanks to quantum superposition. This allows quantum machine learning to solve problems that would take classical computers an impractical amount of time. In theory, QML algorithms could solve certain problems in minutes that would otherwise take years.
2. Improved Computational Efficiency
Quantum ML is also more computationally efficient. Quantum computers require fewer resources to process the same amount of data compared to classical computers. This increased efficiency means that ML models can scale better, handling larger data sets and more complex problems without requiring excessive time or energy.
3. Tackling High-Dimensional Data Sets
High-dimensional data is notoriously difficult for classical systems to handle. With quantum computing’s ability to process vast amounts of data in parallel, QML algorithms can tackle these high-dimensional problems with ease. This advantage is particularly useful in fields such as climate modeling, where the complexity and size of data sets often overwhelm classical systems.
4. Advanced Pattern Recognition
Quantum ML offers more advanced pattern recognition capabilities. Classical ML models, while effective, are limited by their inability to process data at the quantum level. Quantum computers, however, can analyze and detect patterns in quantum states themselves, making QML ideal for fields such as cryptography and drug discovery, where pattern recognition at a subatomic level is necessary.
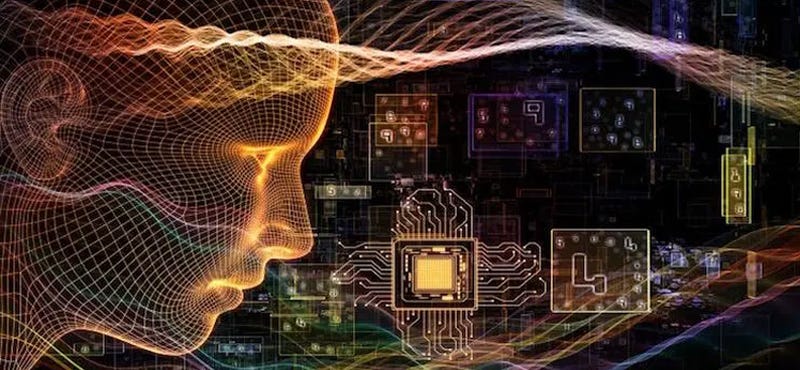
Use Case Studies
1. Quantum-Assisted Drug Discovery
One of the most promising applications of quantum machine learning is in drug discovery. Classical computers struggle with the simulation of molecular interactions, which is essential for understanding how drugs will behave in the human body. Quantum computers, paired with ML algorithms, can simulate these interactions at the atomic level, allowing for faster and more accurate drug discovery.
For example, Cambridge Quantum Computing has developed quantum machine learning techniques that can predict molecular behavior more accurately, drastically reducing the time required to bring new drugs to market. Quantum ML models can simulate the quantum mechanical properties of molecules, which enables more efficient screening of potential drugs.
2. Quantum Finance: Portfolio Optimization
In finance, the goal of portfolio optimization is to find the most efficient allocation of assets to maximize returns while minimizing risk. Quantum machine learning models can solve these optimization problems exponentially faster than classical models. Goldman Sachs has invested heavily in quantum research, particularly in QML algorithms that optimize large portfolios by simulating multiple market scenarios in parallel.
By applying quantum-enhanced ML algorithms, financial institutions are not only speeding up portfolio optimization but also improving accuracy, resulting in more effective investment strategies.
3. Climate Modeling and Quantum Machine Learning
Climate change presents one of the most complex computational challenges due to the vast amount of data and variables involved in accurate modeling. Quantum computers have the potential to process the massive amounts of climate data needed to model weather patterns and predict future climate scenarios with much higher precision.
By leveraging quantum-enhanced ML algorithms, organizations like IBM Quantum are working on simulating climate patterns that would otherwise take too long on classical computers. Quantum ML models can process high-dimensional environmental data, leading to more accurate predictions, which could inform better global climate policies.
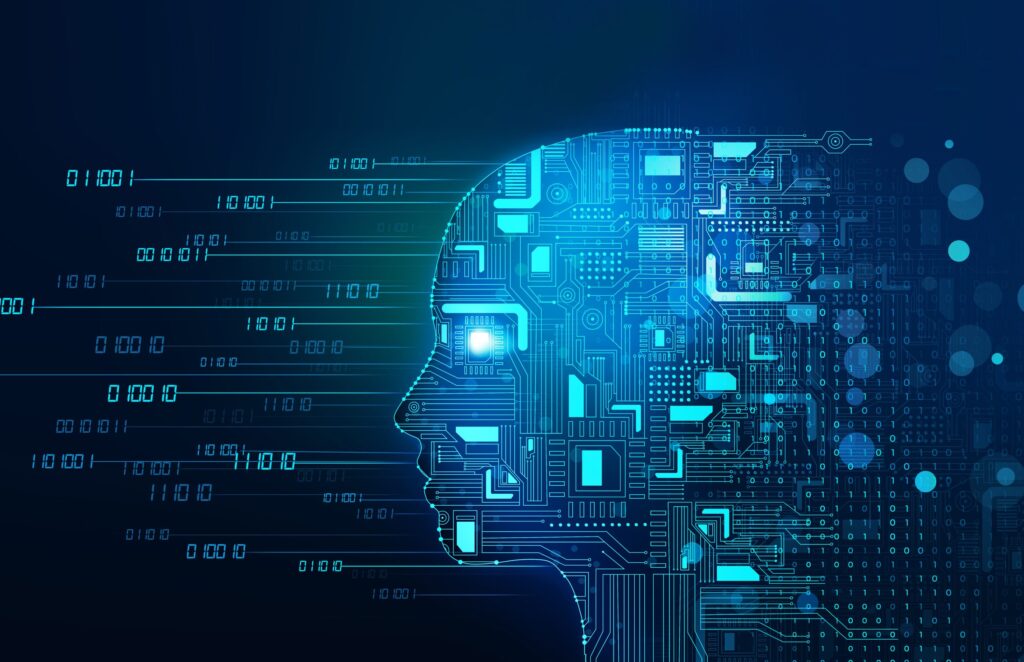
Future Outlook: Quantum Machine Learning Beyond 2024
As quantum technology continues to advance, the applications of quantum machine learning are expected to grow exponentially. Quantum computers are still in the early stages of development, with many technical challenges to overcome, such as error correction and hardware scalability. However, the potential for quantum machine learning is enormous, with industries like healthcare, finance, logistics, and environmental science set to benefit significantly.
1. Breakthroughs in Hardware and Algorithms
Advancements in quantum hardware will play a crucial role in expanding QML’s real-world applications. Companies like Google and IBM are actively developing quantum processors that can handle more qubits and operate with lower error rates. This, combined with breakthroughs in quantum machine learning algorithms, will push the boundaries of what is computationally possible.
2. Hybrid Quantum-Classical Systems
In the short term, hybrid quantum-classical systems are expected to bridge the gap between current quantum hardware limitations and the full potential of quantum computing. These systems will allow us to start using quantum machine learning in practical applications sooner, while quantum computers mature.
Conclusion
The pervasiveness of ML in quantum technology signifies a monumental leap in computational capabilities. From error correction in quantum systems to solving complex optimization problems, the fusion of ML and quantum computing opens new avenues across industries. As both technologies evolve, their synergy will continue to accelerate breakthroughs in science, industry, and society.
Quantum machine learning represents the future of artificial intelligence and computation, and its potential to solve problems currently beyond the reach of classical computers is only beginning to be realized.